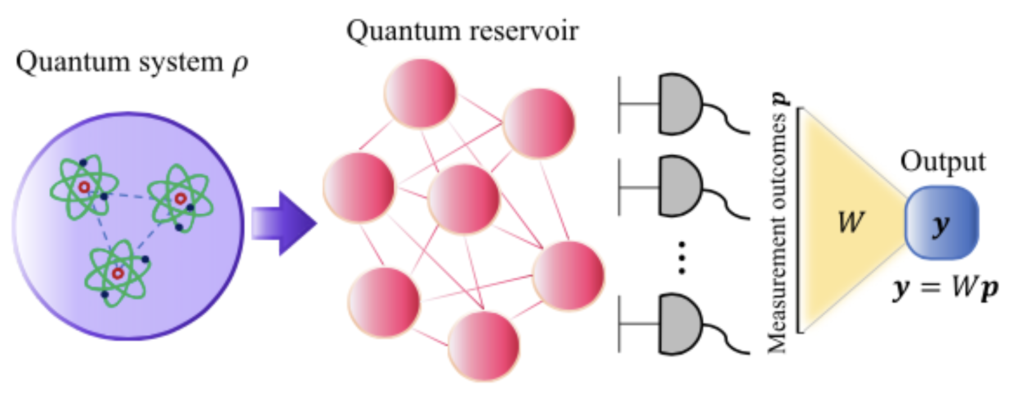
In recent years we have witnessed a growing interest for new quantum computation paradigms, beyond the “standard” quantum gates architectures. In this scenario, Quantum Reservoir Computing (QRC) is an emerging field of quantum neuromorphic computing extending the classical paradigm of reservoir computing to the quantum realm. A primary
characteristic of QRC is its leveraging the dynamics of a complex quantum system, a “reservoir”, to efficiently preprocess input quantum states in a way that allows to then easily recover target features via linear post-processing of the measurement data. Different reservoirs are best suited for different tasks. To process time-dependent signals, a reservoir must be able to hold an internal memory of the inputs seen at previous interactions. By contrast, memoryless reservoirs are sufficient for classification or recognition tasks. These protocols are also known as Extreme Learning Machines – ELM, or QELM for the quantum counterpart. The capabilities of QRC and QELM can be traced back to their exploiting dynamical evolutions exploring Hilbert spaces whose dimension increases exponentially with the number of reservoir constituents. This is determinant to obtain rich temporal dynamical behaviors, frequently achieved by introducing disorder in the interactions between system components.
Some useful references: